Landscape research is based on numerous studies of single processes at single sites, scales, and during certain periods depending from each other. These findings are condensed in terms of general inferences that could be transferred to other regions, scales, and time periods, often via implementation in respective models. However, that approach systematically falls short of grasping unexpected relationships and feedbacks between the realms of different disciplines. Revealing complex feedbacks between different "landscape elements" requires comprehensive data sets that describe various aspects of the same region in a coordinated way. This applies even for data acquisition of very different disciplines in order to enable detecting unexpected relationships. Only then the study region can be characterized in a high-dimensional phase-space (cf., Lischeid et al. 2016), i.e., yielding a comprehensive data cube, that could be analysed with powerful modern data mining approaches. To that end, landscape research in the AgroScapeLab Quillow is based on four major pillars:
I. Monitoring
I. Monitoring
Long-term systematic and continuous measurements constitute the backbone of all research activities in the AgroScapeLab Quillow. These data are made available for other researchers and are complemented by additional data sets provided by external research partners, by authorities, via UAV and satellite remote sensing products, and the like. Monitoring comprises meteorological, hydrological, and biogeochemical data, land management and yield data, surveys of weeds, plant infestations and mycotoxins, bird surveys, etc.
II. Process studies
II. Process studies
Single process studies as well as major projects with numerous partners (e.g., CarboZALF, SoilCan, TEROS, Landscales, BioMove, BIBS) focusing on single aspects of landscape processes benefit from the comprehensive background data. On the other hand, results from these studies help to optimize monitoring and modelling activities.
III. Landscape experiments
III. Landscape experiments
Based on comprehensive background data landscape experiments like in the CarboZALF project can be planned and interpreted.
IV. Modelling and integrated data analysis
IV. Modelling and integrated data analysis
Long-term and consistent multivariate data sets are an ideal basis for developing and testing numerical models as well as for application and further development of modern data mining approaches in order to reveal complex interdependencies in landscapes.
Key components of the current ASLQ strategy include - (i) manipulative landscape experiments,
- (ii) a platform to develop and test Agriculture 4.0 technologies (e.g., robotics, tractor-based sensor networks, geophysics, etc.), and
- (iii) the development of a landscape research approach by bringing together comprehensive datasets from multiple disciplines.
Large heterogeneous datasets (e.g., from satellite and drone remote sensing, tractor-mounted sensors, bat recorders, isotopes, etc.) will be combined to estimate, with high spatial coverage and resolution, the effects of pesticide-free management in the surrounding landscape. Analysis of such datasets requires advanced data mining approaches that consider nonlinearity, nonstationarity, multi-causality, and the spatial dependence in landscape processes. In this way, biodiversity, agricultural, and biogeochemical research can be better interconnected. The ASLQ provides an ideal platform for integrated model development and validation. Urgent and fundamental topics, such as agricultural production in times of climate change and corresponding impacts on the environment, can be addressed following a holistic research approach.
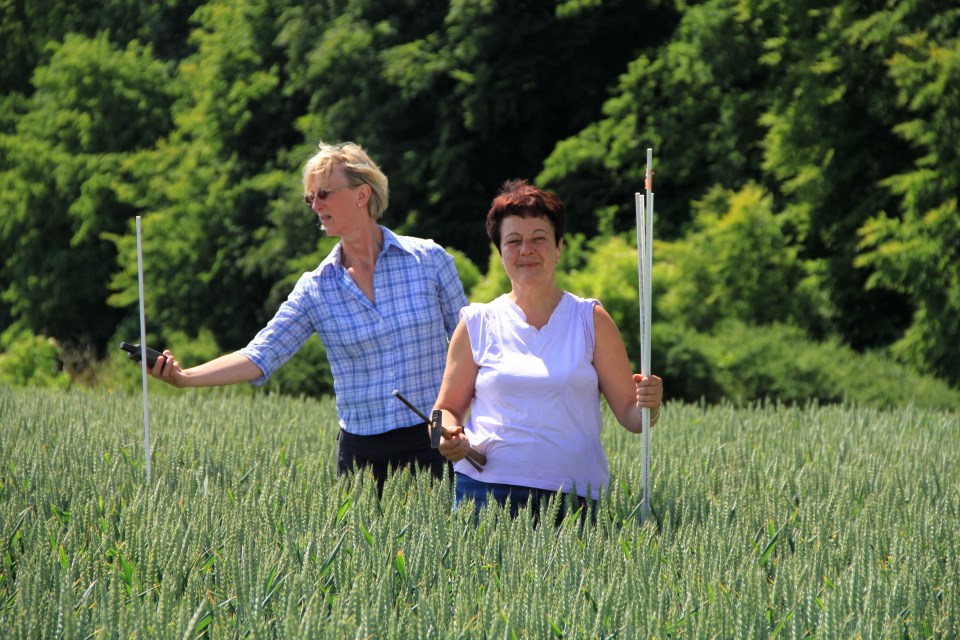 Sampling of phytopathogenic fungi in the field. © Marina Müller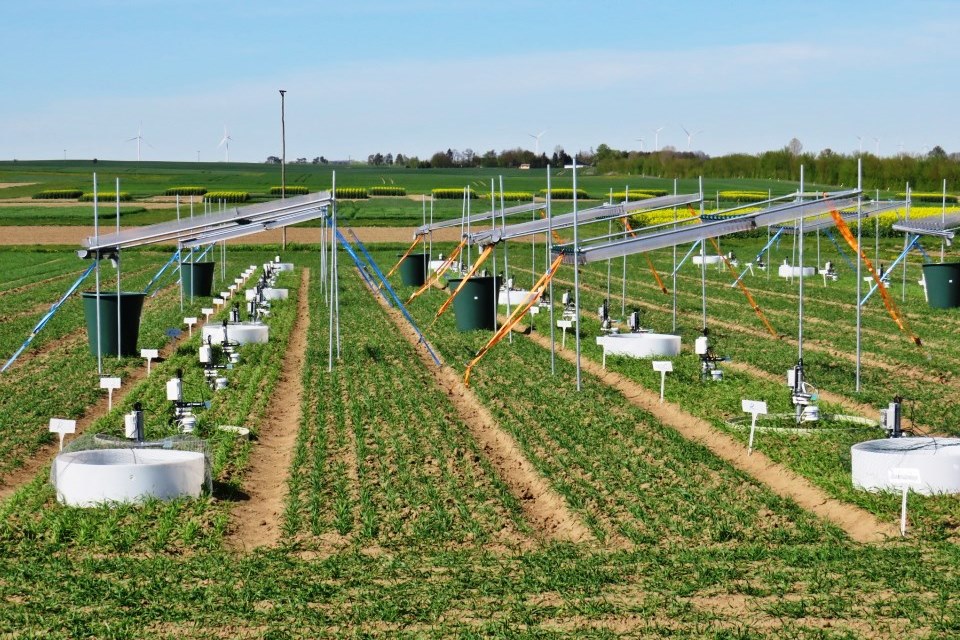 Experimental site of project Volcorn. © Gernot Verch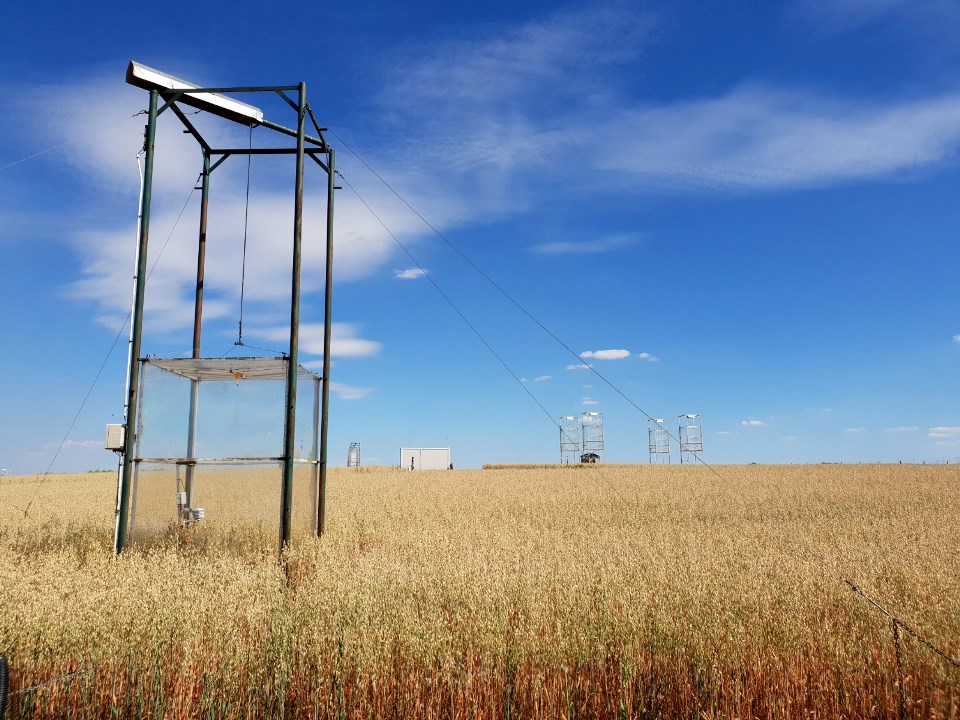 Gas measurement hoods at the Dedelow research station. © Marten Schmidt
References used in text |